Ultrathink: why Claude is still the king
Data Doesn't Lie: Despite Free Alternatives, Developers Pay Premium for Claude's Reliability
OpenRouter has become the de-facto place for new models to be dropped, and the open source community is embracing this practice, with an ever growing number of tokens being sent through them.
What makes OpenRouter valuable isn't just that it provides access to multiple models, but that it gives us visibility into actual usage. Before, we had to rely on company press releases and cherry-picked benchmarks. Now we can see what developers are actually choosing when they build things.For example, we can see lots of new apps using Deepseek's latest v3 model.
Google's token usage is high, but a significant portion is subsidized
We decided to see which is really the favorite model today on the platform; across all apps. On an absolute basis, Google seems to be winning by a lot, but does that mean their models are the best?
If you zoom out, the story is a bit different. Google’s models are genuinely good—I'm not suggesting otherwise—but about ~22% of their total token usage (as of today) is subsidized via free models. This turns out to be a great strategy for getting more usage, but it doesn't tell you much about what models are actually the best.
Free tokens are like free samples at the grocery store. They're good for getting people to try your product, but they don't necessarily indicate preference. What matters more is what people choose when they're spending their own money.
Claude is still the favorite paid model in OpenRouter
Compare this to Anthropic: they have no free models at all. In fact, Claude 3.7 Sonnet is getting used even more than Gemini 2.5 Pro’s free and paid versions combined!
Of course if you’re cash-strapped you’ll use Gemini 2.5 Pro, but you’ll be subject to heavy rate-limiting. So when you get fed up with that, you have to choose: do you pay for the same model you’ve been using, or do you switch to a different model? Among those willing to pay, Claude 3.7 Sonnet is seeing three times as much usage.
Without a doubt the growth of Google has been incredible, and I really like that they're releasing cheap models which perform very well, plus bigger context windows with great retrieval capabilities. But they are only winning if you look at their free models. It'll be really interesting to see if Gemini 2.5's usage stays this high once those free credits dry up.
This reminds me of what happened with cloud providers. AWS wasn't always the cheapest option, but it became the most trusted. When you're building something that matters, you optimize for reliability over cost within reason. The fact that developers are willing to pay more for Claude suggests they're getting something valuable in return.
I think what we're seeing is the beginning of a bifurcation in the market. There will be cost-sensitive applications that use whatever is cheapest (or free). And there will be reliability-sensitive applications that use whatever performs most consistently, even at a premium. This has happened in virtually every technology market as it matures.
What's Anthropic’s secret sauce?
Yesterday, Dario Amodei, the founder of Anthropic published a short article about The urgency of Interpretability, and we couldn't agree more. Interpretability is the science to understand what's really going on inside language models, not just optimizing for benchmark scores but actually figuring out how all the decisions are made.
This focus on interpretability isn't new for Anthropic. They have a deep research tradition in this area going back to Chris Olah's pioneering work. In fact, back in 2021, they created Garcon, a “microscope” to understand what's going on inside LLMs. This tool allowed Anthropic researchers to keep probing the internal workings of these models, access intermediate activations, and modify individual components—essentially giving them x-ray vision into the neural networks.
About a month ago, they published "On the Biology of a Large Language Model", where their interpretability team tackled the fundamental question of how LLMs generate text. They're not just building black boxes; they're trying to understand what's happening inside.
Why does this matter? When you're doing complex tasks like coding, you need models that can follow multiple steps without losing track of what they're doing. Understanding how models work internally lets you improve their reasoning abilities in these multi-step scenarios.
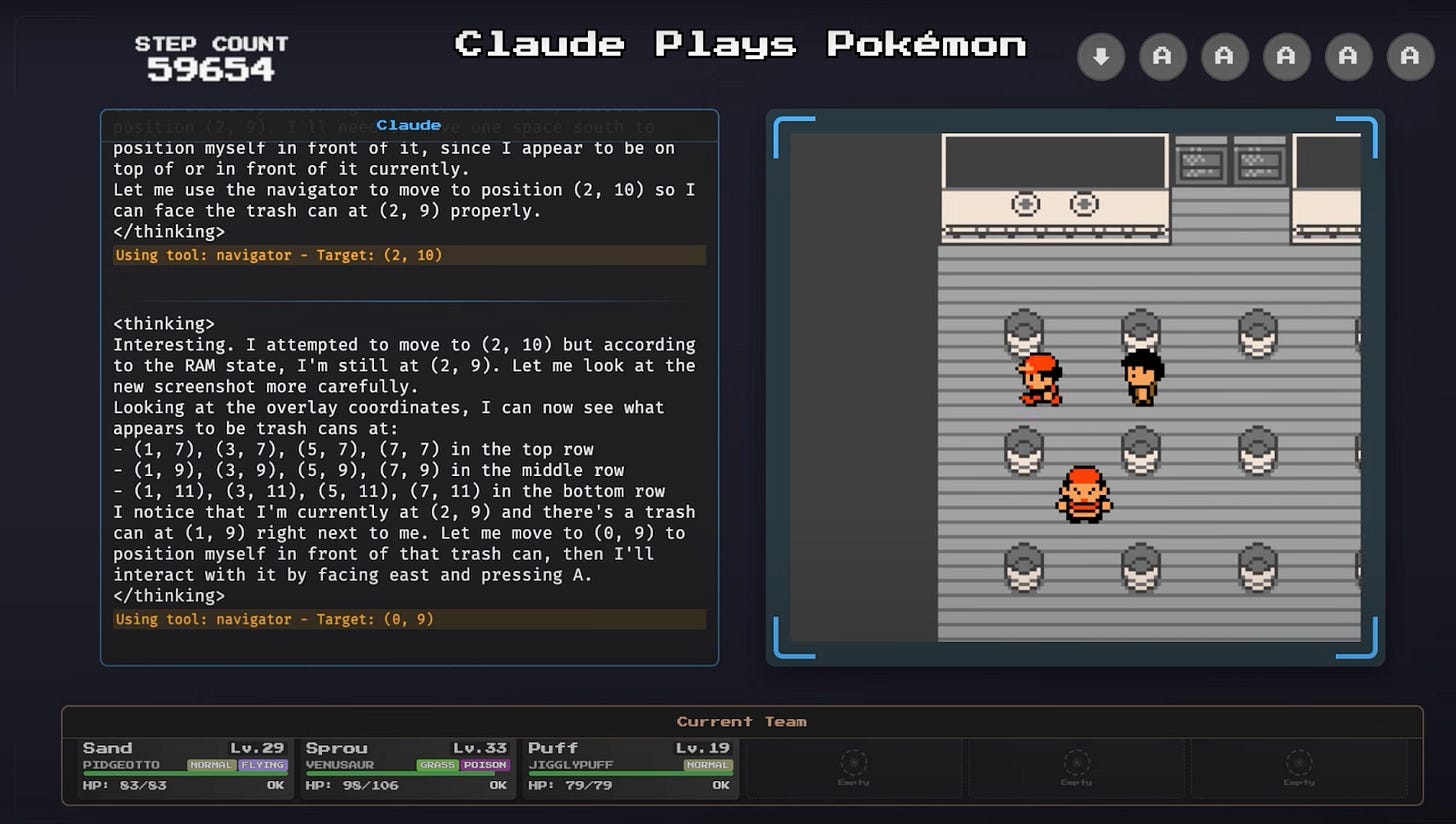
I suspect this deep focus on understanding why their models work, not just that they work, is building the kind of trust and reliability that keeps developers coming back, even when cheaper or free options are everywhere. When you're building something real, predictable, high-quality output often matters more than raw token price. That's where Claude continues to shine.
Most AI benchmarks today measure capabilities in ideal conditions. They don't adequately capture reliability—how consistently a model performs across diverse, real-world scenarios. Anthropic seems uniquely focused on this aspect, which matters enormously in production.
The market is speaking clearly: despite aggressive pricing from competitors and even free alternatives, developers are choosing to pay for Claude. The value gap must be significant enough to overcome price sensitivity.
What we're witnessing is the AI market maturing from novelty to utility. The initial excitement about capabilities is giving way to practical concerns about reliability. This transition happens in every technology market, and as AI becomes more integrated into critical systems, consistency will matter more than raw performance.
The models that win long-term won't necessarily be the ones with the flashiest demos or lowest prices, but those that developers can truly depend on. Right now, Claude seems to understand this better than most—and the usage data suggests developers agree.